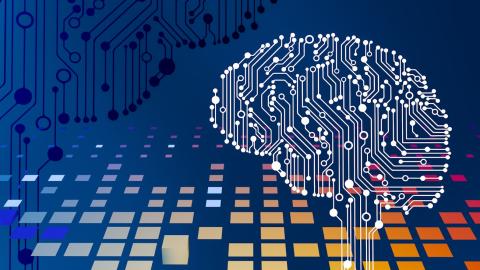
Plasticity of synapses in the brain is responsible for memory, learning and intelligence. Consequently, emulating aspects of biological synaptic plasticity is a fundamental goal in electrical engineering, machine learning and neuromorphic computing. Recently, a new form of synaptic plasticity was discovered in the rodent hippocampus that stores temporally extended memories after just a single trial. Here, we present the first hardware-level emulation of this behavioral timescale synaptic plasticity (BTSP) utilizing a prototypical quantum material, vanadium dioxide (VO2). We apply our circuit design and algorithm to real-time maze solving, which results in rapid learning of an efficient path to reward. Our results open a path to implementation of cutting-edge neuroscience principles in AI hardware with quantum materials.
Alessandro R. Galloni, Yifan Yuan, Minning Zhu, Haoming Yu, Ravindra S. Bisht, Chung-Tse Michael Wu, Christine Grienberger, Shriram Ramanathan, and Aaron D. Milstein